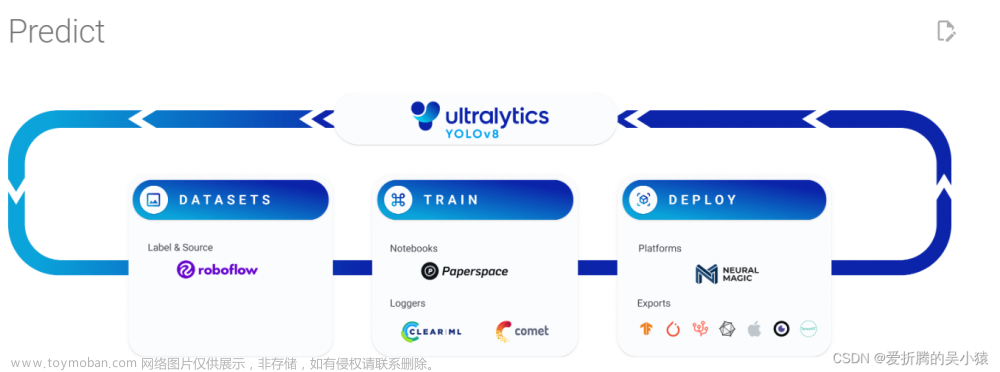
YOLOv8现在可以接受输入很多,如下表所示。包括图像、URL、PIL图像、OpenCV、NumPy数组、Torch张量、CSV文件、视频、目录、通配符、YouTube视频和视频流。表格✅指示了每个输入源是否可以在流模式下使用,并给出了每个输入源使用流模式的示例参数
预测参数
Key | Value | Description |
---|---|---|
source |
'ultralytics/assets' |
source directory for images or videos |
conf |
0.25 |
object confidence threshold for detection |
iou |
0.7 |
intersection over union (IoU) threshold for NMS |
half |
False |
use half precision (FP16) |
device |
None |
device to run on, i.e. cuda device=0/1/2/3 or device=cpu |
show |
False |
show results if possible |
save |
False |
save images with results |
save_txt |
False |
save results as .txt file |
save_conf |
False |
save results with confidence scores |
save_crop |
False |
save cropped images with results |
hide_labels |
False |
hide labels |
hide_conf |
False |
hide confidence scores |
max_det |
300 |
maximum number of detections per image |
vid_stride |
False |
video frame-rate stride |
line_width |
None |
The line width of the bounding boxes. If None, it is scaled to the image size. |
visualize |
False |
visualize model features |
augment |
False |
apply image augmentation to prediction sources |
agnostic_nms |
False |
class-agnostic NMS |
retina_masks |
False |
use high-resolution segmentation masks |
classes |
None |
filter results by class, i.e. class=0, or class=[0,2,3] |
boxes |
True |
Show boxes in segmentation predictions |
下面是每个参数的解释:
-
source
:输入源的目录,可以是图像或视频文件。 -
conf
:目标检测的对象置信度阈值。只有置信度高于此阈值的对象才会被检测出来。默认值为0.25
。 -
iou
:非极大值抑制(NMS)的交并比(IoU)阈值。用于在重叠较大的候选框中选择最佳的检测结果。默认值为0.7
。 -
half
:是否使用半精度(FP16)进行推理。半精度可以减少计算量,但可能会牺牲一些精度。默认值为False
。 -
device
:模型运行的设备,可以是cuda设备(cuda device=0/1/2/3)或CPU(device=cpu)。 -
show
:是否显示检测结果。如果设置为True
,则会在屏幕上显示检测到的对象。默认值为False
。 -
save
:是否保存带有检测结果的图像。如果设置为True
,则会将检测结果保存为图像文件。默认值为False
。 -
save_txt
:是否将检测结果保存为文本文件(.txt)。默认值为False
。 -
save_conf
:是否将检测结果与置信度分数一起保存。默认值为False
。 -
save_crop
:是否保存裁剪后的带有检测结果的图像。默认值为False
。 -
hide_labels
:是否隐藏标签。如果设置为True
,则在显示检测结果时不显示对象标签。默认值为False
。 -
hide_conf
:是否隐藏置信度分数。如果设置为True
,则在显示检测结果时不显示置信度分数。默认值为False
。 -
max_det
:每张图像的最大检测数。如果检测到的对象数超过此值,将保留置信度高低来保留。默认值为300
。 -
vid_stride
:视频帧率步长。默认值为False
,表示使用默认的帧率。 -
line_width
:边界框的线宽。如果设置为None
,则根据图像大小进行自动缩放。默认值为None
。 -
visualize
:是否可视化模型特征。默认值为False
。 -
augment
:是否对预测源应用图像增强。默认值为False
。 -
agnostic_nms
:是否使用类别无关的NMS。默认值为False
。 -
retina_masks
:是否使用高分辨率的分割掩膜。默认值为False
。 -
classes
:按类别过滤结果。可以指定单个类别(例如class=0
)或多个类别(例如class=[0,2,3]
)。默认值为None
,表示不进行类别过滤。 -
boxes
:在分割预测中显示边界框。默认值为True
。
使用Results对象
Results
对象包含以下组件:
Results.boxes
:用于操作边界框的属性和方法的对象
Results.masks
:用于索引掩膜或获取分段坐标的对象
Results.probs
:包含类别概率或逻辑值的张量(tensor)
Results.orig_img
:加载在内存中的原始图像
Results.path
:包含输入图像的路径
result对象默认是torch.Tensor对象,也可以转为其他对象
results = results.cuda()
results = results.cpu()
results = results.to('cpu')
results = results.numpy()
Boxes
Boxes对象可用于索引、操作边界框,并将其转换为不同的格式。Box格式转换结果是缓存的,这意味着每个对象只计算一次,并且这些值将在将来的调用中重复使用。
results = model(img)
boxes = results[0].boxes
box = boxes[0] # returns one box
box.xyxy
Boxes 的属性有
boxes.xyxy # xyxy 形式的目标框, (N, 4)
boxes.xywh # xywh 形式的目标框, (N, 4)
boxes.xyxyn # xyxy 形式的目标框且归一化, (N, 4)
boxes.xywhn # xywh 形式的目标框且归一化, (N, 4)
boxes.conf # 置信度的分数, (N, 1)
boxes.cls # 类别, (N, 1)
boxes.data # 原始目标框参数坐标 (x, y, w, h)、置信度以及类别, (N, 6) or boxes.boxes
Masks
Masks可以被索引、操作修改、将Masks转换为分割结果。也可以缓存段转换操作。
results = model(inputs)
masks = results[0].masks # Masks object
masks.xy # x, y segments (pixels), List[segment] * N
masks.xyn # x, y segments (normalized), List[segment] * N
masks.data # raw masks tensor, (N, H, W) or masks.masks
Probs 置信度
包含了所有类别的置信度
results = model(inputs)
results[0].probs # cls prob, (num_class, )
更多文档可以参考 https://docs.ultralytics.com/reference/yolo/engine/results/
绘制结果
yolov8提供了plot函数绘制结果,可以绘制边框,分割结果,分类结果类别等等。不在需要像yolov5那样直接写后处理nms等,很方便。
res = model(img)
res_plotted = res[0].plot()
cv2.imshow("result", res_plotted)
Argument | Description |
---|---|
conf (bool) | 是否绘制检测置信度得分。 |
line_width (int, optional) | 边界框的线宽。如果为None,则根据图像大小进行缩放。 |
font_size (float, optional) | 文本的字体大小。如果为None,则根据图像大小进行缩放。 |
font (str) | 用于文本的字体。 |
pil (bool) | 是否使用PIL库进行图像绘制。 |
example (str) | 要显示的示例字符串。用于指示输出的期望格式。 |
img (numpy.ndarray) | 绘制到另一个图像上。如果为None,则绘制到原始图像上。 |
labels (bool) | 是否绘制边界框的标签。 |
boxes (bool) | 是否绘制边界框。 |
masks (bool) | 是否绘制掩膜。 |
probs (bool) | 是否绘制分类概率。 |
视频流数据源
以下是使用OpenCV和YOLOv8在视频帧上运行推理的代码。文章来源:https://www.toymoban.com/news/detail-509159.html
import cv2
from ultralytics import YOLO
# Load the YOLOv8 model
model = YOLO('yolov8n.pt')
# Open the video file
video_path = "path/to/your/video/file.mp4"
cap = cv2.VideoCapture(video_path)
# Loop through the video frames
while cap.isOpened():
# Read a frame from the video
success, frame = cap.read()
if success:
# Run YOLOv8 inference on the frame
results = model(frame)
# Visualize the results on the frame
annotated_frame = results[0].plot()
# Display the annotated frame
cv2.imshow("YOLOv8 Inference", annotated_frame)
# Break the loop if 'q' is pressed
if cv2.waitKey(1) & 0xFF == ord("q"):
break
else:
# Break the loop if the end of the video is reached
break
# Release the video capture object and close the display window
cap.release()
cv2.destroyAllWindows()
文章来源地址https://www.toymoban.com/news/detail-509159.html
到了这里,关于YOLOv8预测参数详解(全面详细、重点突出、大白话阐述小白也能看懂)的文章就介绍完了。如果您还想了解更多内容,请在右上角搜索TOY模板网以前的文章或继续浏览下面的相关文章,希望大家以后多多支持TOY模板网!