1写在前面
今天可算把key
搞好了,不得不说🏥里手握生杀大权的人,都在自己的能力范围内尽可能的难为你。😂
我等小大夫
也是很无奈,毕竟奔波霸
、霸波奔
是要去抓唐僧的。 🤐
好吧,今天是词云
(Wordcloud
)教程,大家都说简单,但实际操作起来又有一些难度,一起试试吧。😋
2用到的包
rm(list = ls())
library(tidyverse)
library(tm)
library(wordcloud)
3示例数据
这里我准备好了2
个文件用于绘图,首先是第一个文件,每行含有n
个词汇。🤣
dataset <- read.delim("./wordcloud/dataset.txt", header=FALSE)
DT::datatable(dataset)
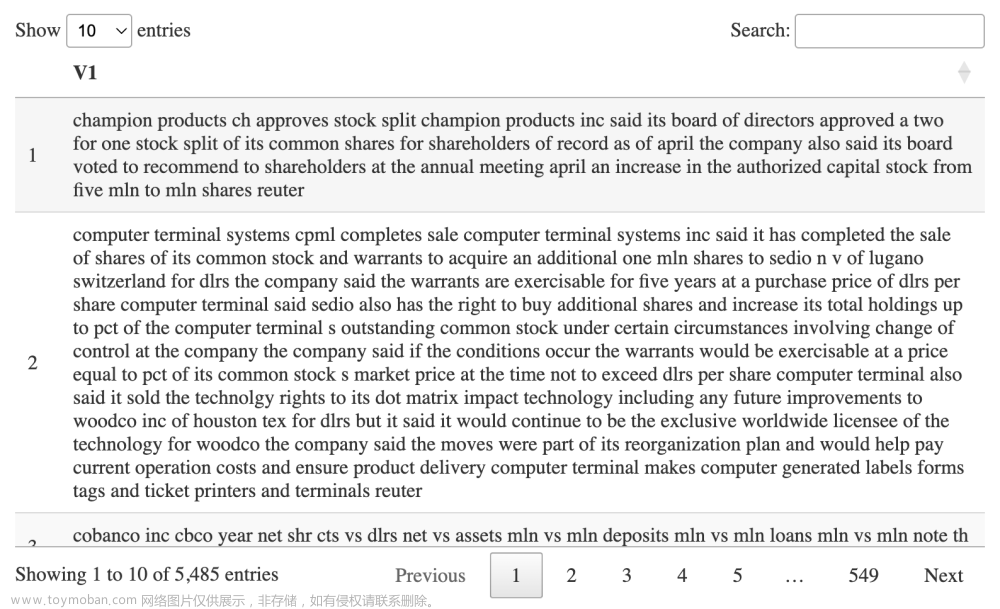
接着是第2
个文件,代表dataset
文件中每一行的label
。🥸
dataset_labels <- read.delim("./wordcloud/labels.txt",header=FALSE)
dataset_labels <- dataset_labels[,1]
dataset_labels_p <- paste("class",dataset_labels,sep="_")
unique_labels <- unique(dataset_labels_p)
unique_labels

4数据初步整理
然后我们利用sapply
函数把数据整理成list
。😘
可能会有小伙伴问sapply
和lapply
有什么区别呢!?😂
ok
, sapply()
函数与lapply()
函数类似,但返回的是一个简化的对象,例如向量或矩阵。😜
如果应用函数的结果具有相同的长度和类型,则sapply()
函数将返回一个向量。
如果结果具有不同的长度或类型,则sapply()
函数将返回一个矩阵。😂
dataset_s <- sapply(unique_labels,function(label) list( dataset[dataset_labels_p %in% label,1] ) )
str(dataset_s)
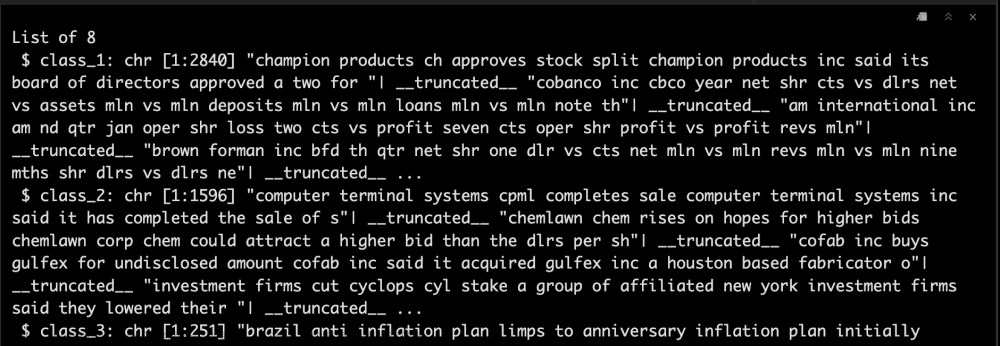
5数据整理成Corpus
接着我们把上面整理好的list
中每个元素都整理成一个单独的Corpus
。🤩
dataset_corpus <- lapply(dataset_s, function(x) Corpus(VectorSource( toString(x) )))
然后再把Cporus
合并成一个。🧐
dataset_corpus_all <- dataset_corpus
6去除部分词汇
修饰一下, 去除标点、数字、无用的词汇等等。😋
dataset_corpus_all <- lapply(dataset_corpus_all, tm_map, removePunctuation)
dataset_corpus_all <- lapply(dataset_corpus_all, tm_map, removeNumbers)
dataset_corpus_all <- lapply(dataset_corpus_all, tm_map, function(x) removeWords(x,stopwords("english")))
words_to_remove <- c("said","from","what","told","over","more","other","have",
"last","with","this","that","such","when","been","says",
"will","also","where","why","would","today")
dataset_corpus_all <- lapply(dataset_corpus_all, tm_map, function(x)removeWords(x, words_to_remove))
7计算term matrix并去除部分词汇
document_tm <- TermDocumentMatrix(dataset_corpus_all)
document_tm_mat <- as.matrix(document_tm)
colnames(document_tm_mat) <- unique_labels
document_tm_clean <- removeSparseTerms(document_tm, 0.8)
document_tm_clean_mat <- as.matrix(document_tm_clean)
colnames(document_tm_clean_mat) <- unique_labels
# 去除长度小于4的term
index <- as.logical(sapply(rownames(document_tm_clean_mat), function(x) (nchar(x)>3) ))
document_tm_clean_mat_s <- document_tm_clean_mat[index,]
head(document_tm_clean_mat_s)
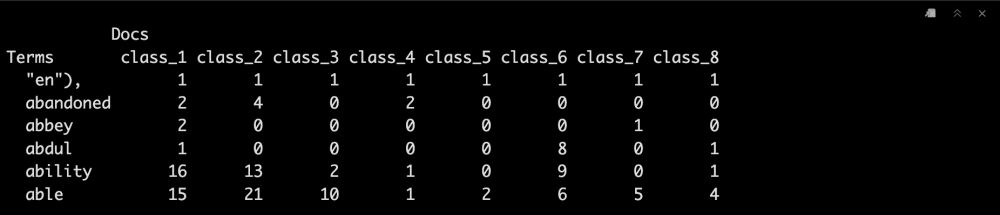
8可视化
8.1 展示前500个词汇
comparison.cloud(document_tm_clean_mat_s,
max.words=500,
random.order=F,
use.r.layout = F,
scale = c(10,0.4),
title.size=1.4,
title.bg.colors = "white"
)
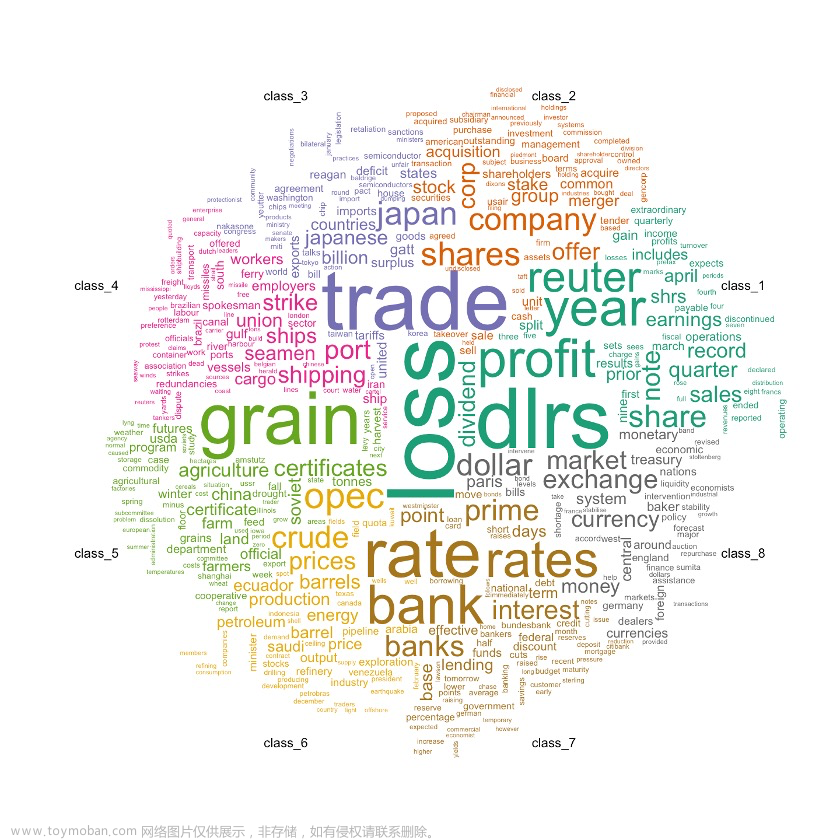
8.2 展示前2000个词汇
comparison.cloud(document_tm_clean_mat_s,
max.words=2000,
random.order=F,
use.r.layout = T,
scale = c(6,0.4),
title.size=1.4,
title.bg.colors = "white"
)
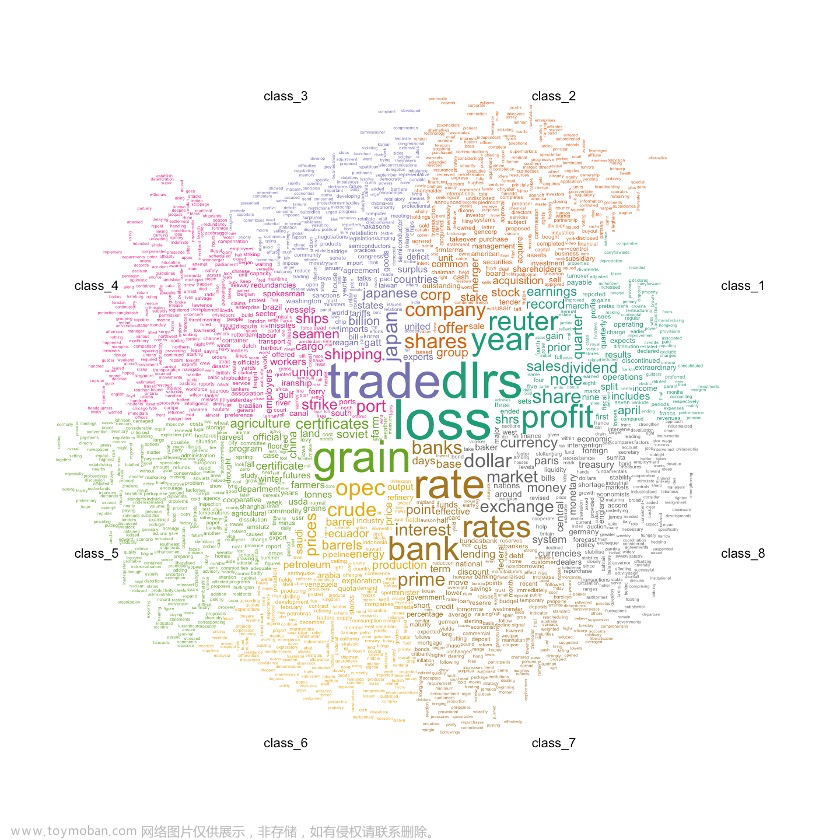
8.3 展示前2000个common词汇
commonality.cloud(document_tm_clean_mat_s,
max.words=2000,
random.order=F)
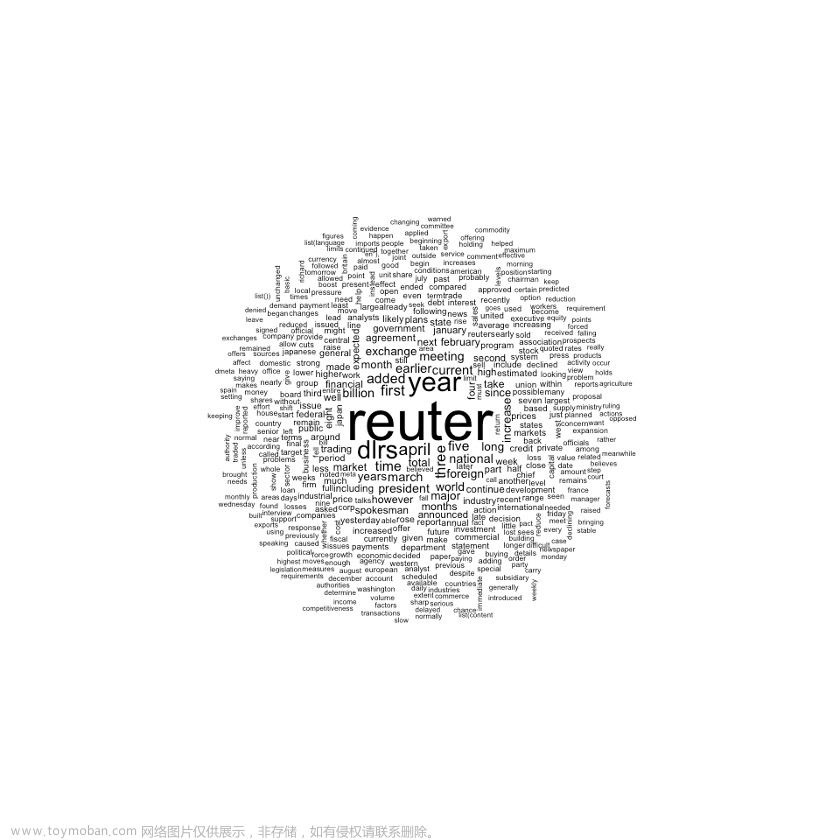

点个在看吧各位~ ✐.ɴɪᴄᴇ ᴅᴀʏ 〰
📍 🤩 LASSO | 不来看看怎么美化你的LASSO结果吗!?
📍 🤣 chatPDF | 别再自己读文献了!让chatGPT来帮你读吧!~
📍 🤩 WGCNA | 值得你深入学习的生信分析方法!~
📍 🤩 ComplexHeatmap | 颜狗写的高颜值热图代码!
📍 🤥 ComplexHeatmap | 你的热图注释还挤在一起看不清吗!?
📍 🤨 Google | 谷歌翻译崩了我们怎么办!?(附完美解决方案)
📍 🤩 scRNA-seq | 吐血整理的单细胞入门教程
📍 🤣 NetworkD3 | 让我们一起画个动态的桑基图吧~
📍 🤩 RColorBrewer | 再多的配色也能轻松搞定!~
📍 🧐 rms | 批量完成你的线性回归
📍 🤩 CMplot | 完美复刻Nature上的曼哈顿图
📍 🤠 Network | 高颜值动态网络可视化工具
📍 🤗 boxjitter | 完美复刻Nature上的高颜值统计图
📍 🤫 linkET | 完美解决ggcor安装失败方案(附教程)
📍 ......文章来源:https://www.toymoban.com/news/detail-660426.html
本文由 mdnice 多平台发布文章来源地址https://www.toymoban.com/news/detail-660426.html
到了这里,关于Wordcloud | 风中有朵雨做的‘词云‘哦!~的文章就介绍完了。如果您还想了解更多内容,请在右上角搜索TOY模板网以前的文章或继续浏览下面的相关文章,希望大家以后多多支持TOY模板网!